Pipeline release! nf-core/ampliseq v2.13.0 - Ampliseq Version 2.13.0!
Please see the changelog: https://github.com/nf-core/ampliseq/releases/tag/2.13.0
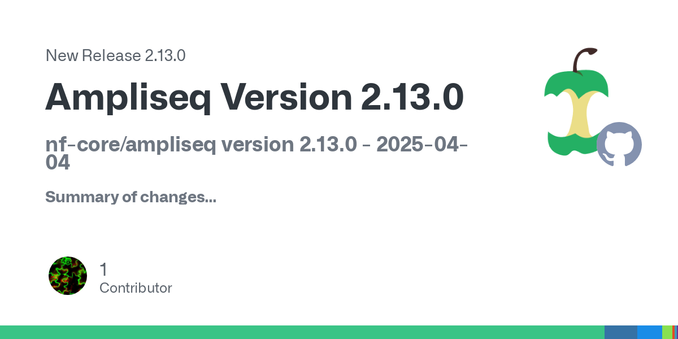
Pipeline release! nf-core/ampliseq v2.13.0 - Ampliseq Version 2.13.0!
Please see the changelog: https://github.com/nf-core/ampliseq/releases/tag/2.13.0
Pipeline release! nf-core/mag v3.4.0 - mag 3.4.0 - Green Gecko!
Please see the changelog: https://github.com/nf-core/mag/releases/tag/3.4.0
Cloud computing: where you're not just a bioinformatician, you’re also the finance manager, compliance officer, and IT support, all while carrying a hefty dose of mental fatigue. I miss those PhD days with a inhouse HPC when all you had to do was sbatch every parameter combo under the sun to test your research question… then complain about queue times and who was clogging the damn cluster with their BLAST jobs
#bioinformatics
The joys of windows line endings creeping into your Linux workflows... #bioinformatics
Test your #coding skills by solving these daily #bioinformatics challenges
biocode - Daily Programming Challenges
https://www.biocodedaily.com/
#Genetic data repo #OpenSNP to self-destruct before authoritarians weaponize it
The reason, according to #bioinformatics researcher Bastian Greshake Tzovaras, one of the founders of the project, is the dissolution of genetic testing biz #23andMe and the potential weaponization of genetic data by #farright #authoritarian regimes.
https://www.theregister.com/2025/04/01/opensnp_shutdown/
On Monday I received this email, sad news:
#cipres #philogeny #biology #bio #genetic #bioinformatics #informatics #taxonomy #science #sci #philogenetics #treeoflife #CIPRES #populationgenetics #code #coding
I'm thinking of submitting an Archaeal RNAseq project here.
Anyone around here in touch with someone at Ultima Genomics? Their sequencing submission web form might have a permission issue :/
asking for a friend: do you know any database/framework to visualize RNAseq data ? (just like https://github.com/broadinstitute/gnomad-browser for WGS)
I've seen this trend myself in the #Bioinformatics #Genomics #NGS community: a few still retweet on the other place, but most original posts are on #Bluesky
RE: https://bsky.app/profile/did:plc:lf4a2b6djtuv5l3lz7ffsyv4/post/3llesxcmuvj2g
Big shoutout to the folks at NCBI GEO. They have been doing an incredible job managing enormous amounts of gene expression and related biomedical data for many, many years They do it efficiently and very, very well. I am so grateful: both when I download data published by others and when I submit my own data. You are true #bioinformatics heroes doing priceless and highly impactful work!
#NCBI #nih
#Genomics
#Bioinformatics
#Academia
Beware US academic coders. If you have collaborators contributing code from a sanctioned region, your repos may be locked.
See https://mastodon.social/@organicmaps/114155428924741370
The kicker is that the US is leaning authoritarian and all in on censorship. If Canada or Mexico become sanction targets and you have code pushes from there, Microsoft will lock you out of your GitHub accounts.
Want to improve your genomic variant calling pipeline? Join our webinar to learn about ncbench, a powerful benchmarking tool. We'll also cover other essential genomic benchmarking topics. Register for free: https://t1p.de/o4al3 #bioinformatics #genomics #variantcalling #ncbench
Does anyone have any posts or past toots they could reshare about how they have setup or are working within a team in #bioinformatics, #genomics, #research etc. things that how worked or haven’t worked, organising and sharing projects and code, notes and documentation, collaboration and communication, software, network and local or cloud compute arrangement. Always find reading others experiences insightful and beneficial.
How does everyone organise all their projects on #GitHub within their teams, like projects into groups or topics? naming schemes or have you switched to #GitLab for this feature?
#research #genomics #bioinformatics
#nfcore "For more transparency and easier management of the proposals, all ideas and concepts for new pipelines will instead be submitted as GitHub issues on a new 'proposals' repository:"
6/n If you want to support our work, you could become part of our #community efforts by a Rechenkraft.net #charity #membership and add your ideas to ours. ;-)
#DIYbio #lab #instruments #OpenSource #chemistry #bioinformatics #CompChem #AugmentedReality #MINT #Schule #Lehre #STEM #education #TiltFive